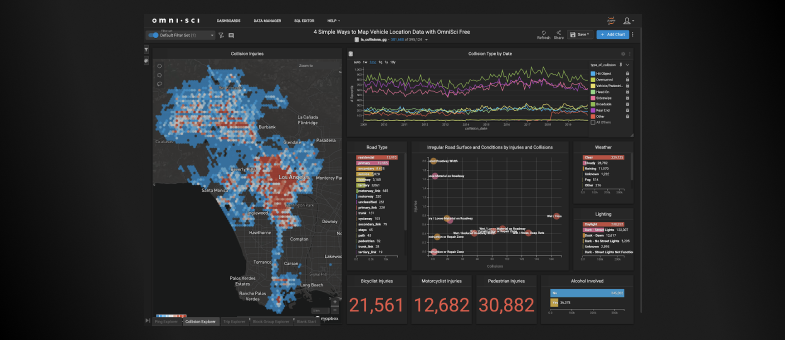
4 Simple Ways to Map Vehicle Location Data with OmniSci Free
Try HeavyIQ Conversational Analytics on 400 million tweets
Download HEAVY.AI Free, a full-featured version available for use at no cost.
GET FREE LICENSENOTE: OMNISCI IS NOW HEAVY.AI
Take a second and think of the noisiest, most unreadable map you've ever seen.
The one that immediately comes to mind for me was in 2015. I worked for a GIS software company at the time and was visiting one of the Big 3 automotive manufacturers in Detroit.
A solution engineer I was presenting with had just got his hands on the now famous NYC Taxi dataset and put together a quick demo to show how we could map what we thought to be big data.
The result was both impressive and comical—one giant, yellow blob that covered the island of Manhattan.
Fast forward six years and growth in safety and security services, rising demand for raw diagnostic data, and increasing adoption of connected car services have made massive vehicle location datasets even more unwieldy.
An average connected vehicle generates over 25 gigabytes of data every hour—a conservative estimation from industry experts.
Making sense of heavy, spatially enabled data like this requires sound exploration, which starts with placing things in a geospatial context.
Let's take a look at 4 simple ways to map vehicle location data with OmniSci Free, a full-featured version of our analytics platform available for use at no cost:
- Pointmap with Density Gradient
- Aggregated by Bin
- Enriched Streets
- Summarized by Census Block Group
1. Pointmap with Density Gradient
The first and most straightforward approach to mapping vehicle locations is the pointmap, which plots geographic measures like latitude and longitude.
To get started, you select your desired data source and the columns that represent your geographic measures. For this example, we use 500+ million anonymized vehicle location points within the greater Los Angeles metro and their X and Y fields to satisfy the required inputs.
The pointmap, by default, presents each record as an individual point on the map and enables point autosize and density gradient, two key features that make this workflow super easy.
Point autosize adjusts each point's size as you pan and zoom the map, and the density gradient performs a count aggregation by pixel that allows you to normalize the count and apply a sweet data visualization color palette to the data.
Here we use a diverging blue-to-yellow color palette against Mapbox's dark basemap to make the densest pockets of vehicle locations pop.
What could have looked like that giant, yellow blob I saw back in 2015 is now an insightful map that can act as the backbone of an exploratory, visual analytics dashboard.
2. Aggregated by Bin
Extracting actionable insights from vehicle location data demands an understanding of where things went wrong just as much as the status quo.
For the next example, we import and synthesize over ten years of California Statewide Integrated Traffic Records System (SWITRS) collision data to plot using OmniSci Free's geo heatmap feature.
The geo heatmap displays aggregate values by geographic location and allows users to compare information by dynamic polygons called bins.
Similar to the pointmap, you set your data source and geographic dimensions.
One additional parameter you have to set is the color measure which, in this case, represents the collision variable we want to aggregate and display. We are most interested in understanding where the largest concentration of injuries occurred, leading us to select the sum count of injuries.
We can choose to display the bin shape as hexagons or squares and adjust the bin pixel size to smaller or larger bins to increase or decrease the geo heatmap's granularity.
Applying a bin size of nine, layer opacity of 75, and a diverging blue-to-red color palette puts the finishing touches on this beautiful and informative map that highlights troublesome collision hotspots throughout the metro.
3. Enriched Streets
Geo heatmap charts are excellent for higher zoom levels but tend to lose their effectiveness when zooming in tight to a neighborhood or street. An easy solution to this problem is to employ multi-layer geo charts and scale-dependent zooming.
In this example, we add a layer to the geo heatmap chart from above. The second layer consists of street centerline buffers enriched with collision summary statistics. A choropleth chart plots these polygons by accepting a geometry field and a color measure that tells the map what summary statistic we want to use to symbolize the buffers.
We create a similar symbology to the geo heatmap by visualizing the aggregated number of injuries that occurred on each road segment, set a layer opacity of 75, and choose a diverging blue-to-red color palette.
In addition to these settings, we match the border color to fill and include a popup that displays:
- The road name
- Road type
- The number of injuries
- And the average party count of collisions on that road segment
When we navigate back to the master chart, we see the layers, their draw order, and the zoom levels at which they're visible.
We want to present the new streets layer to the viewer once we zoom past a certain level on the map. Zoom levels 0 through 11 are given to the geo heatmap and 11 through 22 to the streets. The new streets layer instantaneously replaces the geo heatmap once we zoom past 11.
We can apply this method to eight layers and any combination of map charts, depending on the server memory and processing capacity, to construct user-friendly maps and dashboard experiences.
4. Summarized by Census Block Group
Finally, let's take a look at another exciting use of the choropleth map chart by revisiting the 500+ million anonymized vehicle location points we used in our pointmap example.
This time we've summarized the vehicle locations by census block group to create a true classic—the color-coded, thematic map.
Our data source is the enhanced block groups, and its geometry field is the base omnisci_geo type. We stylize the polygons by the total distance traveled per block group and give them a popup that shows the total number of vehicle observations and time spent in each geography.
The Mapbox dark basemap is our choice to compliment the blue-gray to aqua graduated color palette. We match the polygons' border with the fill to eliminate any clutter and turn the opacity down to 70—chef's kiss!
There you have it, four simple ways to map vehicle location data with OmniSci Free! Try for yourself, download OmniSci Free today
These techniques are a great way to start mapping your in-house vehicle location data, premium location data services, or an entirely different use case altogether.
Be sure to stay up-to-date with the OmniSci blog and look out for a deep dive into the spatial analysis methods we used to enrich the street buffers and census block groups with vehicle location and crash data variables.
Share any cool maps and dashboards you create with us on LinkedIn, Twitter, or our Community Forums!